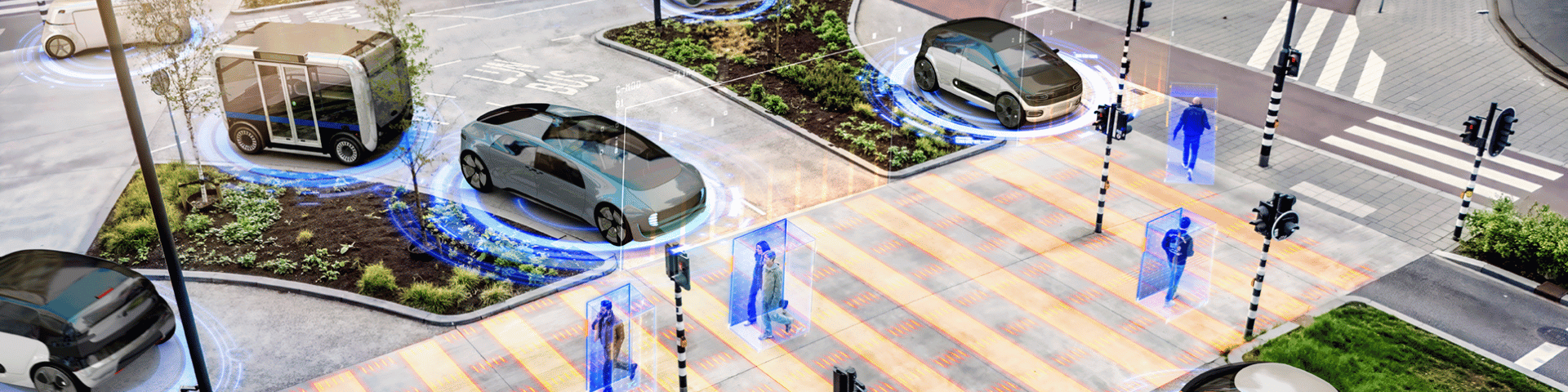
Automated vehicle technology for public roads
Automated driving technology is steadily progressing, with promising applications being rolled out across various sectors. Hub-to-hub autonomous trucking offers significant economic potential. Level 3 self-driving passenger cars enable drivers to use travel time more effectively. And automated public transport systems promise higher availability at lower costs.
Current challenges
However, significant challenges related to safety, reliability, and regulatory frameworks still hinder the large-scale implementation of autonomous driving.
Before widespread adoption of self-driving vehicles can be considered, it's essential to ensure they are completely reliable and capable of handling all possible road scenarios.
Our vision
Through the development of components and architectures that prioritise safety by design, our aim is to enhance the overall safety of the entire vehicle.
It's critical to utilise all available information that a vehicle can gather about its driving environment and internal state, including reliability estimates, to enhance the safety of self-driving vehicles.
Our solutions
The advancement of automated driving relies on reliable localisation, which can be achieved through the use of multiple new sensor inputs, sophisticated sensor signal fusion techniques, and online estimation of localisation integrity. TNO offers proven, beyond the state-of-the-art technology building blocks that enhance the capabilities of self-driving systems to accurately navigate diverse road environments, improving overall safety and reliability
Autonomous vehicles, without input or control from the driver, must continuously assess and adapt to changing road conditions. To ensure safe and trustworthy estimations, self-driving vehicles can utilise and combine various sources of information.
Human drivers are trained to adjust their driving style for safe handling in various conditions, considering factors like tyre wear and weather. They use visual cues and experience to assess road conditions and determine appropriate braking distances.
In autonomous vehicles, information about tyre condition, road conditions, and weather can be gathered using algorithms and sensors. This data, combined with external inputs like real-time vehicle-to-vehicle data and meteorological information, serves as crucial input for safe autonomous driving.
TNO is actively researching advanced sensory systems and algorithms to enhance vehicle safety through several innovation programs. One recent focus area is optimising braking distance by incorporating tyre intelligence into advanced anti-lock brake systems (ABS). Beyond ABS, TNO envisions various promising applications for tyre information, such as in safety-critical automated systems like 'Automated Evasive Steering.' With detailed tyre data, these systems can more accurately assess when to brake or swerve in response to potential accidents, ultimately enhancing vehicle safety.
How can we teach self-driving vehicles to adequately deal with the incredible complexity of our traffic and take adequate decisions in every situation? To teach self-driving vehicles to navigate safely and responsibly, TNO applies an innovative approach focused on context-aware driving behaviour and motion planning using machine reasoning.
The design of self-driving vehicles faces a significant challenge known as combinatorial explosion, where the multitude of situational factors and their combinations make it impractical to explicitly define required behaviours for every scenario. The sheer number of possible combinations is overwhelming, and traditional software development approaches are not scalable for addressing this complexity and iteratively improving the system.
Machine learning, while powerful, lacks guarantees that specific behaviours – like adhering to traffic laws – are explicitly accounted for in all situations. Moreover, machine learning-based behaviours can be difficult to reason about or explain in terms of safety across all scenarios. This presents a critical challenge in ensuring the reliability and safety of self-driving vehicle systems.
To address this challenge, TNO is developing a unique and innovative solution based on machine reasoning. The goal is to create a behaviour specification approach that accounts for all factors of the driving context, such as other road users, road layout, traffic rules, weather conditions, and the capabilities of the automated vehicle. This solution should be understandable, verifiable, and explainable by humans, and specified behaviours should translate seamlessly into actual motion planning for the automated vehicle.
Sensor perception alone is insufficient for self-driving vehicles to accurately understand the world around them. While hybrid systems that combine sensor data with external communication can be more accurate, they are not 100% reliable. TNO is addressing critical cybersecurity and trust challenges within the automotive domain by collaborating with partners to establish a safe and trustworthy digital infrastructure.
Intelligent Speed Assist (ISA) highlights the limitations of relying solely on sensory information. Camera-only systems often provide inaccurate speed limit information, whereas hybrid systems incorporating external map-based data are more reliable. Similarly, roadworks warnings and traffic light status information benefit from external communication with the digital infrastructure. However, even with hybrid systems, reliability is not guaranteed as external information can be incorrect or outdated.
In this innovation programme, TNO is focused on addressing the root causes of incorrect information from the digital infrastructure. The challenge is navigating the complexity of the data chain involving multiple parties such as OEMs, road authorities, telecom providers, and data providers. Ensuring the security and reliability of each element in this chain is critical to prevent the transmission of unreliable information to vehicles, which could lead to unsafe situations. TNO's efforts aim to improve the integrity and security of data transmissions within this complex ecosystem.
Therefore, it's crucial for vehicles to identify and assess external communication, including the quality of the data and the reliability of its source and connection. At TNO, we are developing an automotive trust domain framework that contributes to European standards by evaluating the quality of information across the entire data chain. Leveraging our unique expertise in safety engineering, automotive ICT, data, and cloud technologies, TNO is actively working to establish a safe and trusted digital infrastructure for automated driving.
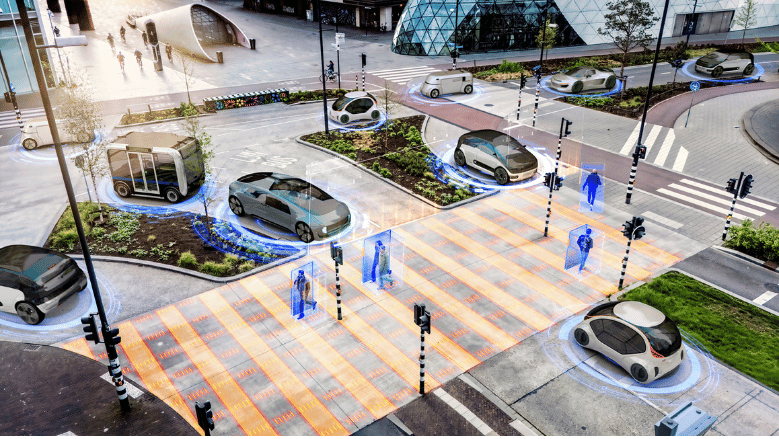
DITM: Digital Infrastructure for Future-proof Mobility
Get inspired
Getting on board with autonomous transport? Five things you need to know
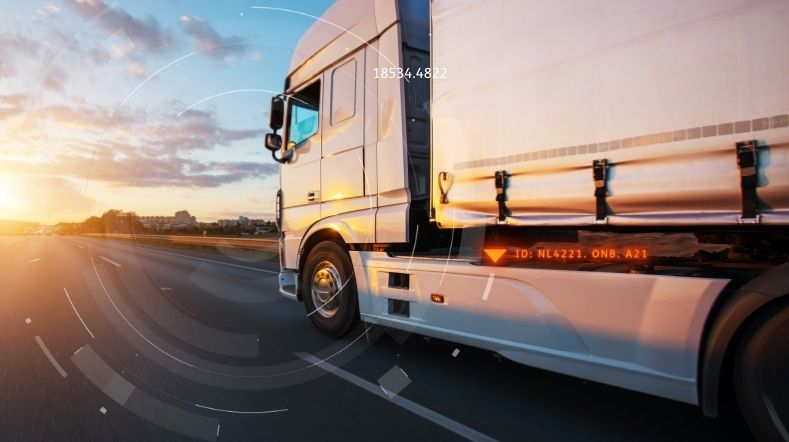
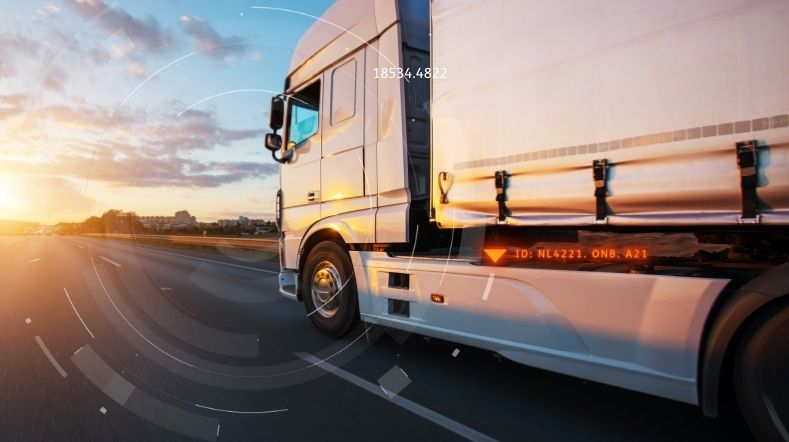
Connected mobility
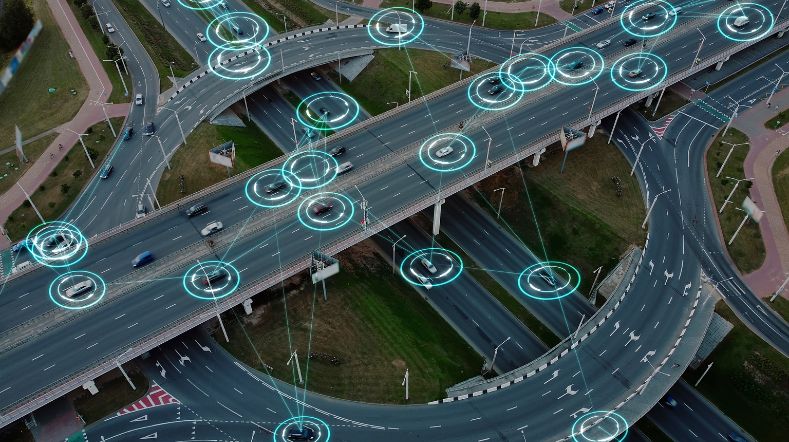
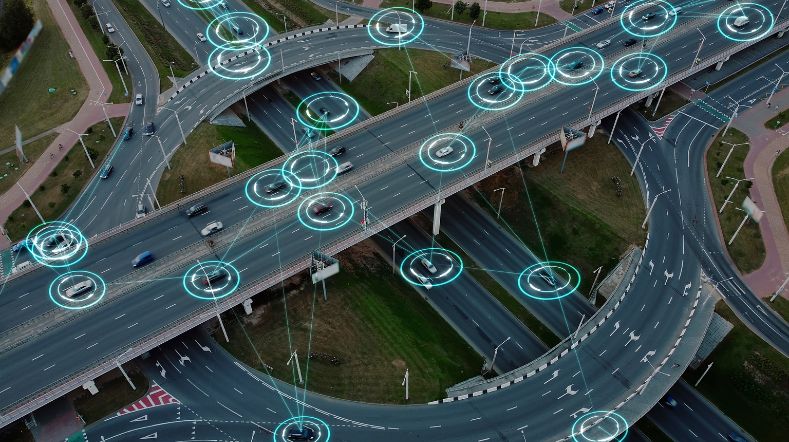
Working on safer self-driving cars
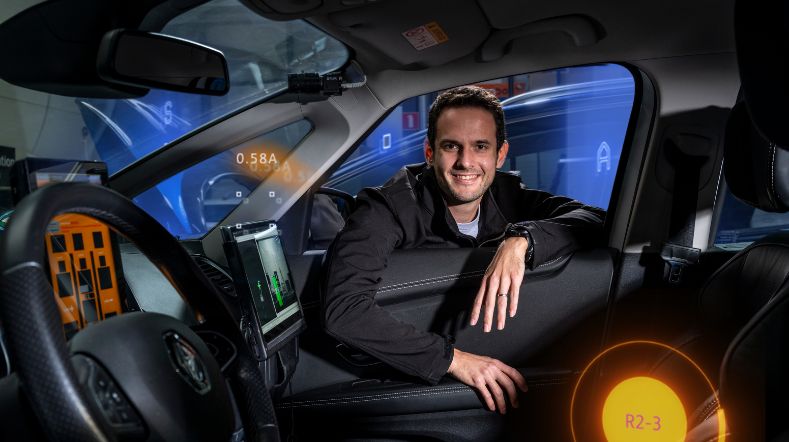
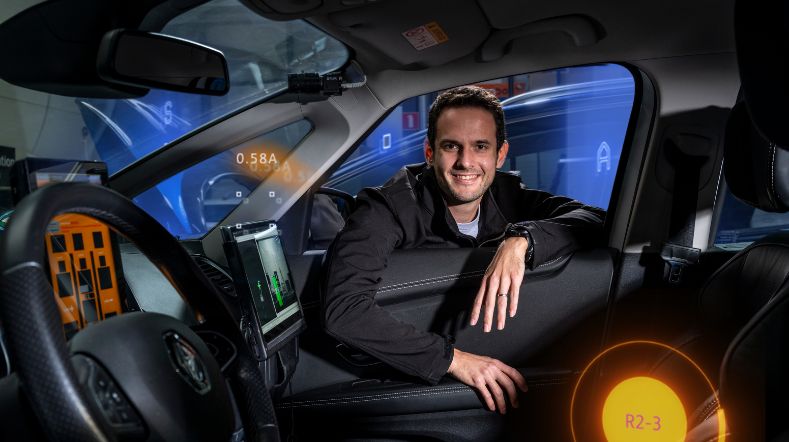
Goodyear and TNO further integrate tyre and vehicle technology to mitigate crashes in adverse weather
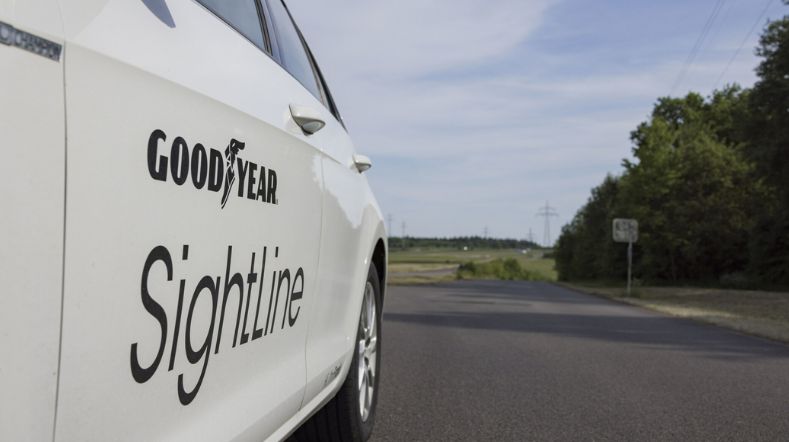
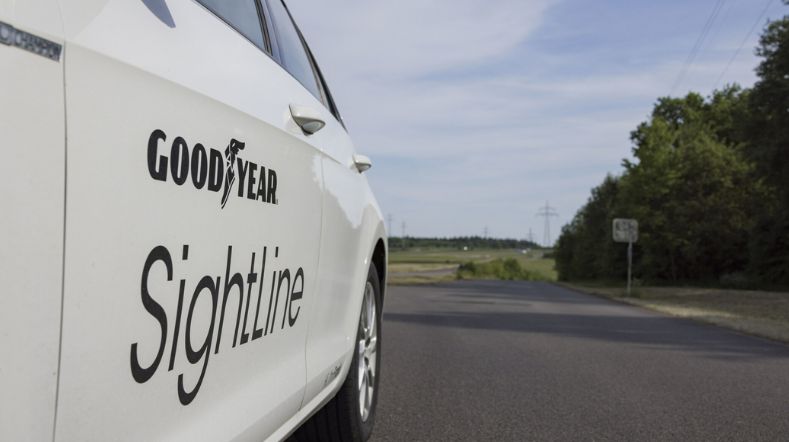
TNO CEYAS offers logistics partners advantage yard automation
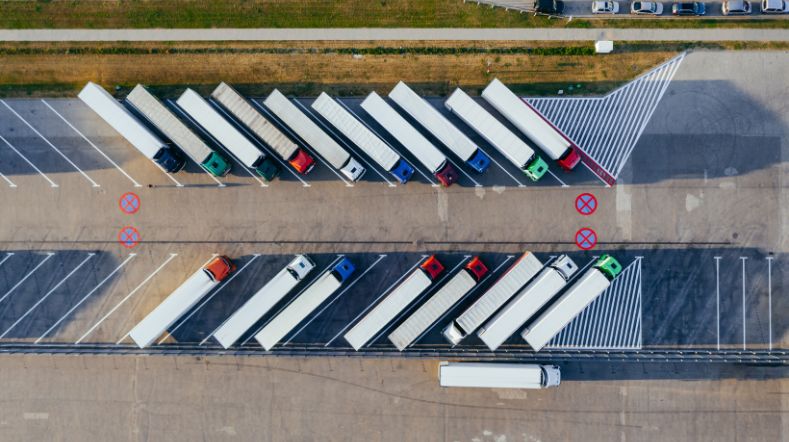
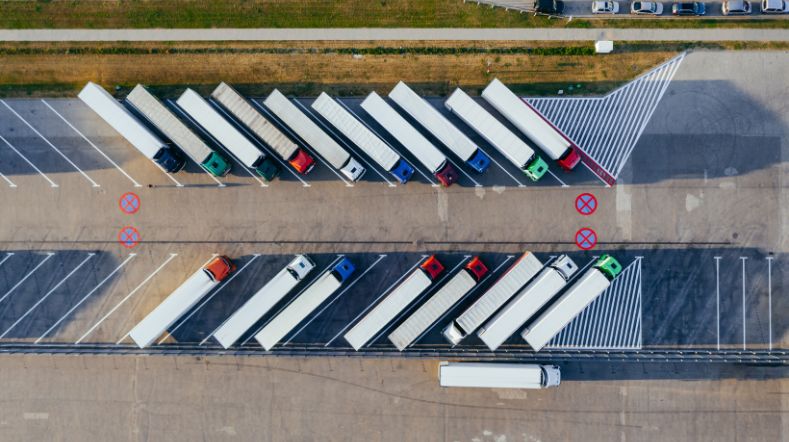