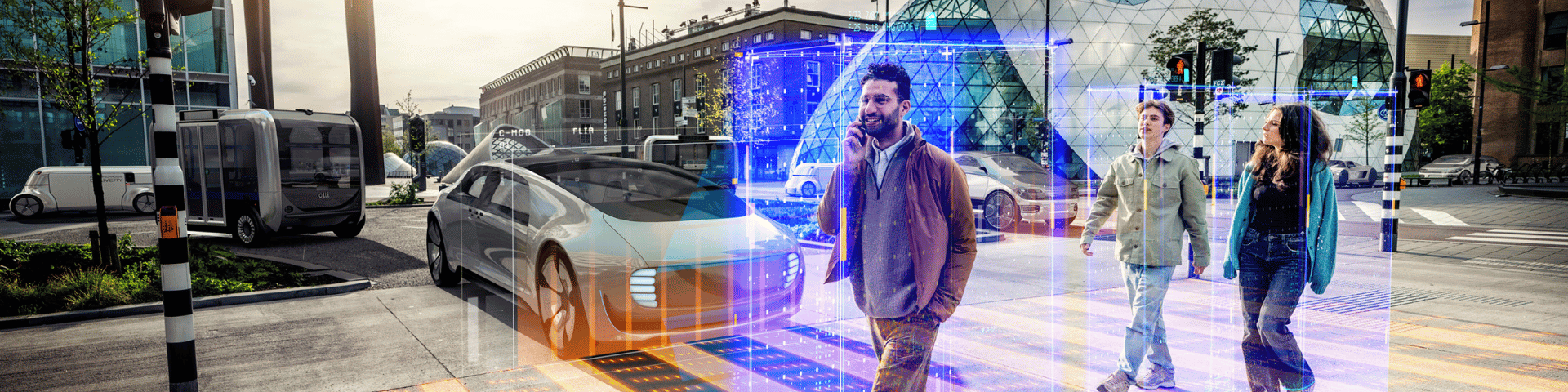
Safety assessment of automated driving
Automated driving's future relies on robust safety validation of vehicles. Reliable safety assessments are crucial before releasing and deploying automated vehicles on public roads to ensure safe operation in all traffic situations, contributing to society's sustainable wellbeing and prosperity.
Current challenges
Automated driving technology has advanced significantly, incorporating sophisticated sensors, AI, and other technologies to handle complex driving situations. However, the key challenge remains proving that these complex systems can interact safely with their environment at all times. This is a core challenge of safety assessment that cannot be fully addressed through real-world driving tests alone.
The use of AI components poses a specific challenge in safety assessment. Machine learning components, often black box functions, lack the ability to reason about their correctness. Furthermore, machine learning technology is predominantly applied in perception stacks, which must handle a vast array of scene variations, adding to the complexity of ensuring safety and reliability.
In addition to technical challenges, compliance with regulations and standards presents another hurdle. Automated driving regulations and standards are often considered "open norms," lacking agreed-upon interpretations or practical implementations. This uncertainty creates challenges for both industry stakeholders and regulators.
Our vision
To ensure comprehensive safety assessment, it's crucial to decompose risks by their sources. By combining knowledge about the system and its architecture with real-world data and simulations, we can estimate the quantitative contribution of different risk sources to overall safety. This approach is essential for determining whether safety requirements are met with reasonable efforts, within reasonable timeframes.
TNO’s thorough and systematic approach extends beyond standards of functional safety (ISO 26262) and safety of intended functionality (ISO/DIS 21448:2021 SOTIF) or regulations like R157 ALKS with the aim to offer practical interpretations of these standards and regulations. Our scientifically grounded methodologies provide a basis for safety assessments by vehicle manufacturers and for regulatory type approval.
Our solutions
StreetWise: Scenario based safety assessment for automated driving
TNO StreetWise is an advanced method for capturing and describing real-world driving conditions and their variations. It uses this data to build a scenario database that enables scenario-based testing and validation of automated driving systems. By leveraging public road driving data for scenario identification and test case generation, StreetWise ensures that virtual test scenarios closely resemble real-world driving conditions. This approach is instrumental in advancing safe automated driving technology and quantifying safety risks.
Safety of AI
To effectively evaluate the performance of automated driving systems that incorporate AI components, TNO is building a comprehensive probabilistic model, based on extensive real-world data and insights in the system’s architecture.
Get inspired
Connected mobility
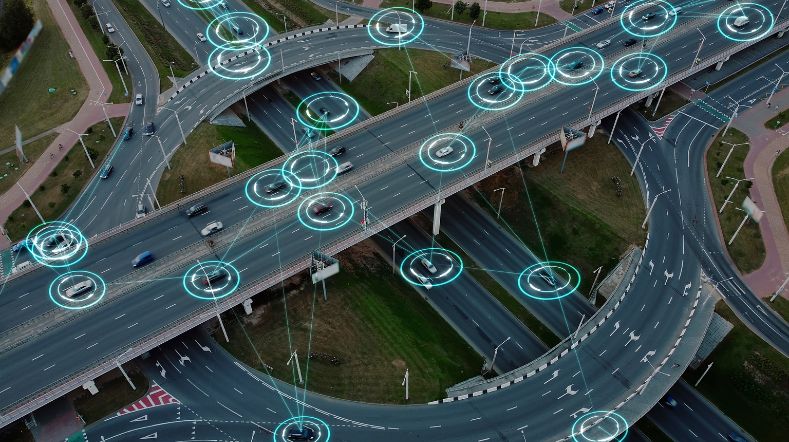
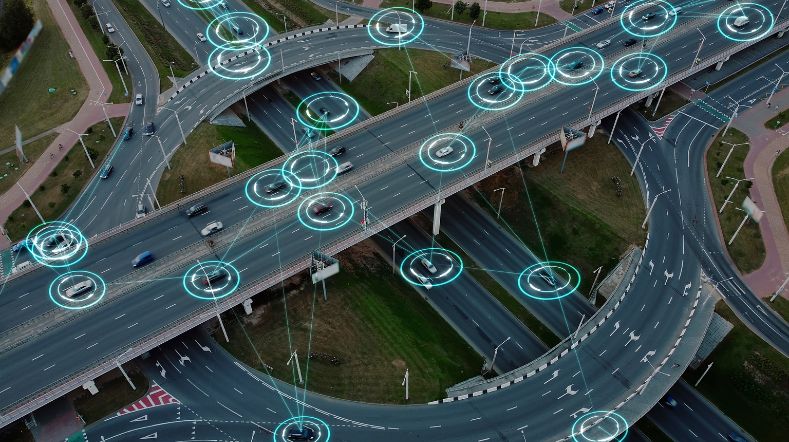
Working on safer self-driving cars
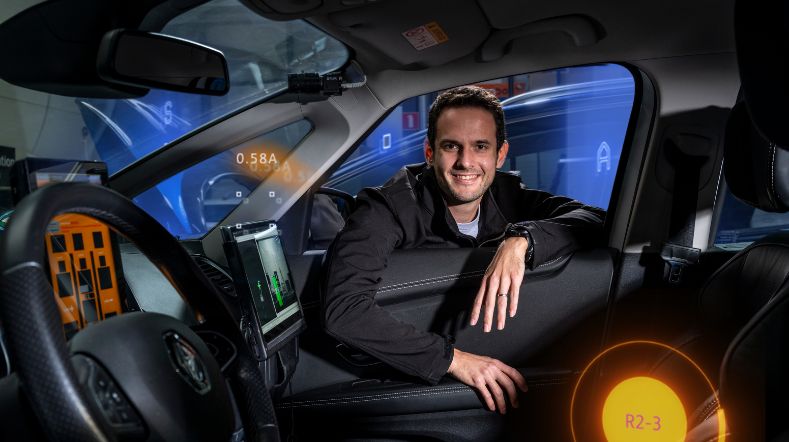
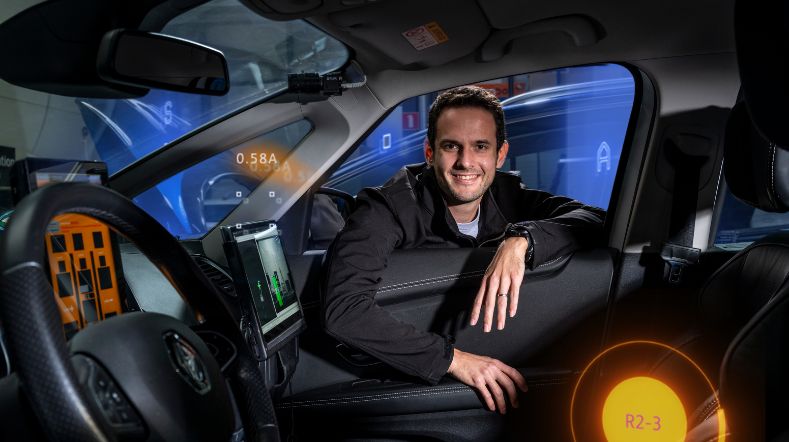
Goodyear and TNO further integrate tyre and vehicle technology to mitigate crashes in adverse weather
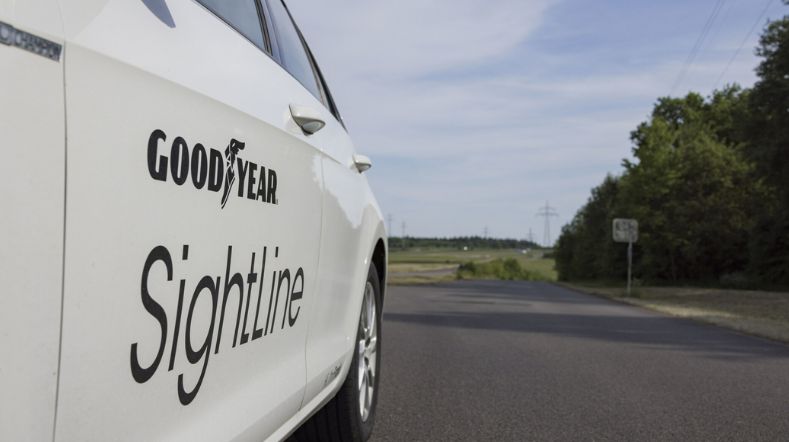
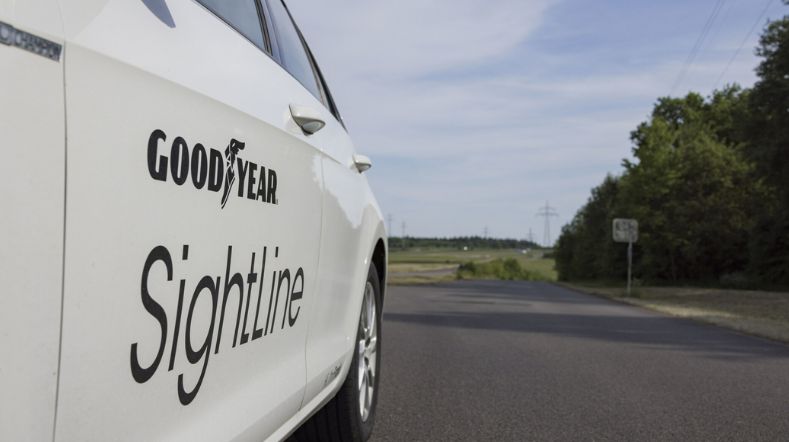
TNO CEYAS offers logistics partners advantage yard automation
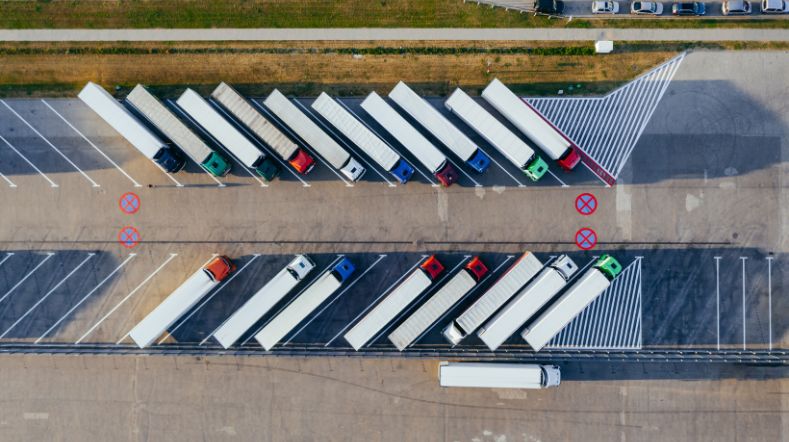
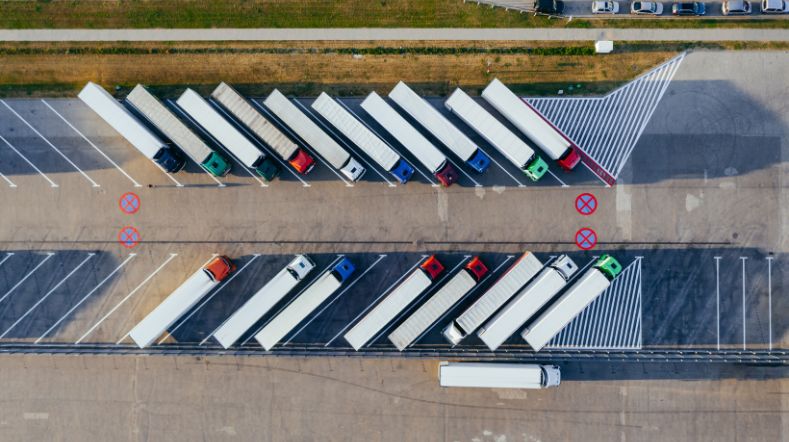
TNO and Japanese counterpart AIST plan to expand cooperation
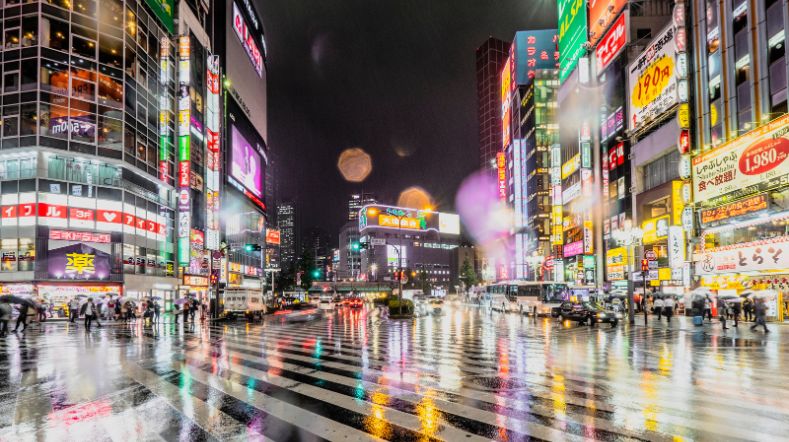
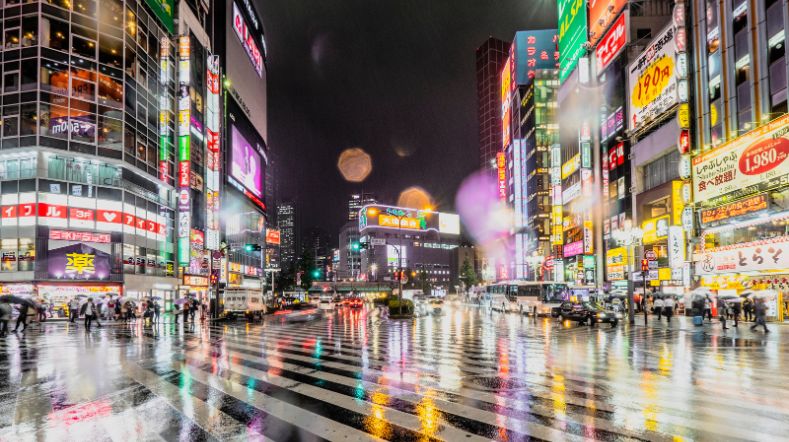