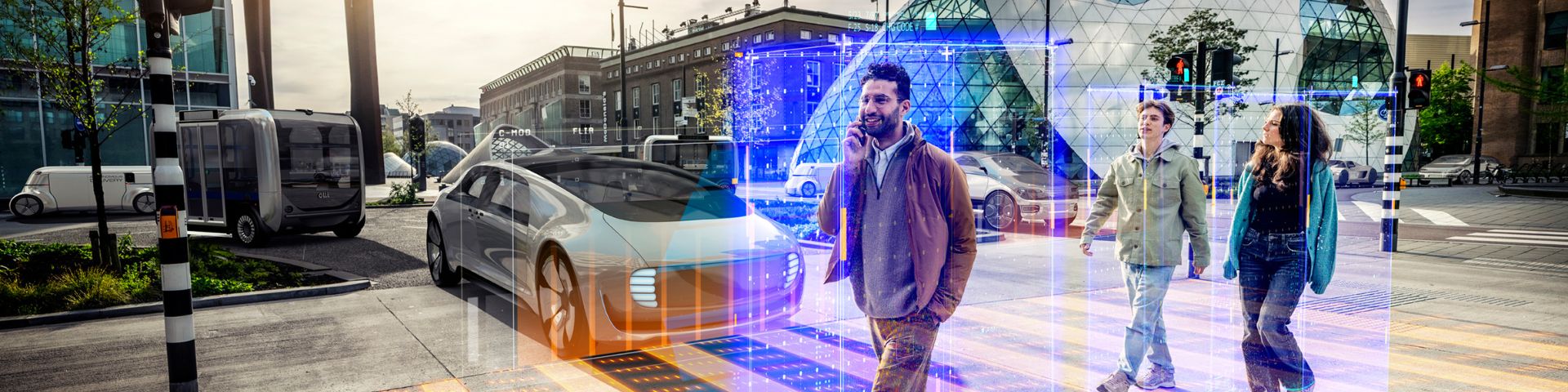
Combining strengths of AI for next generation autonomous driving systems
Incidents and accidents with self-driving vehicles are all over the media. Is it time to say goodbye to the automated driving dream, or can we still turn the tide? TNO scientist Jan-Pieter Paardekooper is optimistic and at the same time realistic about the automated future: ‘We have to teach autonomous vehicles how to reason, it’s the only way to make it happen.’
Loss of trust
In recent years, we have witnessed a shift in sentiment on the subject of automated driving. The initial enthusiasm and optimism among both the public and industry have been replaced by scepticism and pessimism.
Jan-Pieter Paardekooper knows why: ‘High expectations didn't materialise, and predictions had to be repeatedly adjusted. Confidence in automated technology has also been severely impacted by serious accidents resulting in injuries and fatalities, particularly in the US, where regulations for deploying automated vehicles are less stringent compared to other parts of the world.’
‘Following numerous incidents involving partially automated vehicles and autonomous taxis, there is a growing call for stricter federal legislation for autonomous vehicles. The loss of trust in autonomous driving among the general public is understandable. Nevertheless, at TNO we remain optimistic about the potential of self-driving vehicles.’
Meet the expert on 11 July: AI, the solution and the challenge?
AI is a very powerful solution for complex tasks in an automated vehicle. The safety and system engineering challenges for vehicles with AI components are however still an open question. TNO illuminates the underlying issues and the direction for solutions. Meet Jan-Pieter Paardekooper (TNO).
AI technology for self-driving vehicles
The reason for this positive outlook lies in TNO’s innovative take on AI technology for self-driving vehicles. Paardekooper explains, ‘Supervised learning is currently the most popular machine learning technique. Algorithms like deep neural networks analyse data to identify patterns based on labelled examples. As the algorithm processes more data, its decision-making abilities improve and become more refined.’
‘This approach to AI, seen in technologies like ChatGPT, and image generation (e.g., Midjourney), has been highly successful due to the abundance of data and advancements in computing power. Therefore, learning from data has surpassed symbolic AI, which relies on logic and mathematics to define rules. At TNO, we believe in combining the strengths of both learning and symbolic AI for the next generation of autonomous driving systems.’
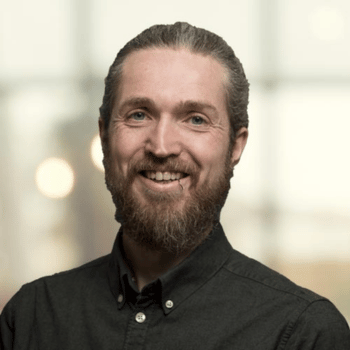
‘At TNO we believe in combining the strengths of both learning and symbolic AI for the next generation of autonomous driving systems.’
Learn how to reason
Current autonomous vehicles use neural networks and data to learn how to drive safely. Paardekooper: ‘This means that the AI tries to understand traffic rules and safe driving behaviour through exposure to countless driving situations on the road. Over time, the system becomes proficient in understanding and responding to most traffic scenarios.’
‘However, if an unknown situation arises, the vehicle may not know how to respond and come to a complete stop or, worse, make the wrong decision and cause an accident. This is inevitable because driving skills cannot be learned solely through accumulating kilometres. Neural networks cannot learn the 'why' behind the examples (no causality), making it impossible for these algorithms to deduce traffic rules from data alone.’
‘Their inability to reason or reflect on their behaviour autonomously becomes evident in complex, unfamiliar situations. Therefore, it is essential for the system to also learn the underlying rules. Essentially, we are teaching autonomous vehicles how to reason.’
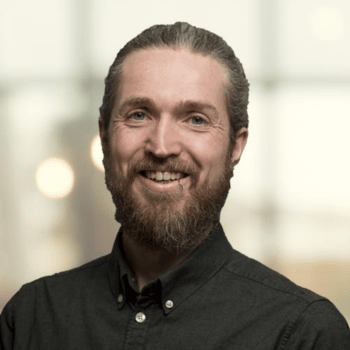
‘By incorporating reasoning rules, we can analyse what occurred in case of an incident and why it happened.’
Black box
One of the significant benefits of adopting this hybrid approach to AI, that combines both learning and symbolic AI, is the ability to understand how autonomous vehicles make decisions. Jan-Pieter Paardekooper explains, ‘With current systems, this understanding is not feasible; it's like dealing with a black box. By incorporating reasoning rules, we can analyse what occurred in case of an incident and why it happened. In other words, as developers, we can learn from the vehicle's behaviour and enhance the system.’
Here's how it works: the vehicle utilises sensors, cameras, and lidars to perceive its surroundings. Through machine learning-based object recognition, it discerns the position of objects and ongoing events. Furthermore, a machine reasoning step generates a risk field by analysing the relevance of general driving rules, as defined by the developer, in conjunction with the observed scenario. This allows the system to continuously assess the risk of various traffic scenarios and deal with them in a safe, legal, ethical and social manner.
Paardekooper: ‘For instance, when overtaking a cyclist, the vehicle takes a wider path compared to manoeuvring around a stationary object, because the system is taught through a driving rule that a collision with the cyclist would cause more harm than collision with a stationary object. Or when a vehicle is driving in a lane marked with a continuous line, the system can reason to cross that line in an emergency manoeuvre around an obstacle in order to avert an accident.’
‘In other words, it knows that avoiding an accident is more important than strictly adhering to the traffic law – something most drivers would do as well. Previously, vehicles would struggle with such contradicting behaviour specifications and are likely to behave unpredictably in such scenarios. Without reasoning capabilities, achieving autonomous driving is unattainable.’
Two-way assessment strategy
Meanwhile, at the Automotive Campus in Helmond, TNO has put theory into practice by conducting tests with industry partners on closed roads. ‘The initial results from testing our hybrid AI system in various scenarios are very promising,’ says Jan-Pieter Paardekooper. ‘The technology is impressive, and at TNO, we believe it can be a game-changer. However, to safely deploy it on the road, we must ensure it can handle any situation.’
Therefore, TNO advocates a two-way development and assessment strategy that combines a safety-by-design approach on a components level, with a wide range of safety assessment methodologies. ‘This comprehensive approach to safety assessment is essential for ensuring the reliability and readiness of autonomous driving technology for real-world deployment. If we succeed in this,’ Paardekooper concludes, ‘I am cautiously optimistic about the future of automated driving.’
Meet the expert on 11 July: AI, the solution and the challenge?
AI is a very powerful solution for complex tasks in an automated vehicle. The safety and system engineering challenges for vehicles with AI components are however still an open question. TNO illuminates the underlying issues and the direction for solutions. Meet Jan-Pieter Paardekooper (TNO).
Get inspired
AI in training: FATE develops digital doctor's assistant
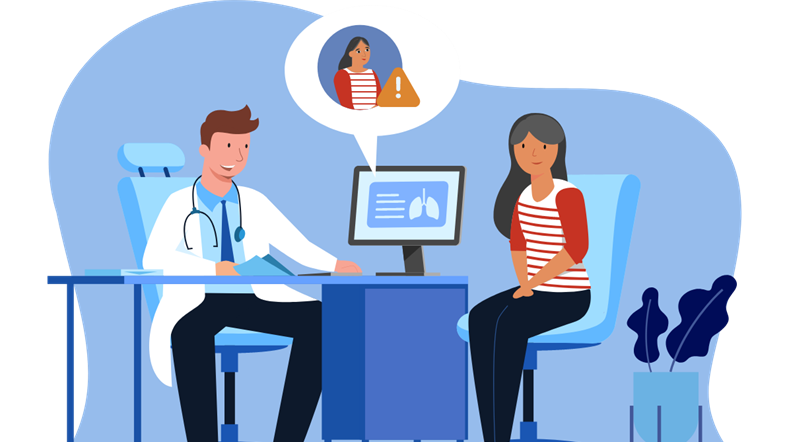
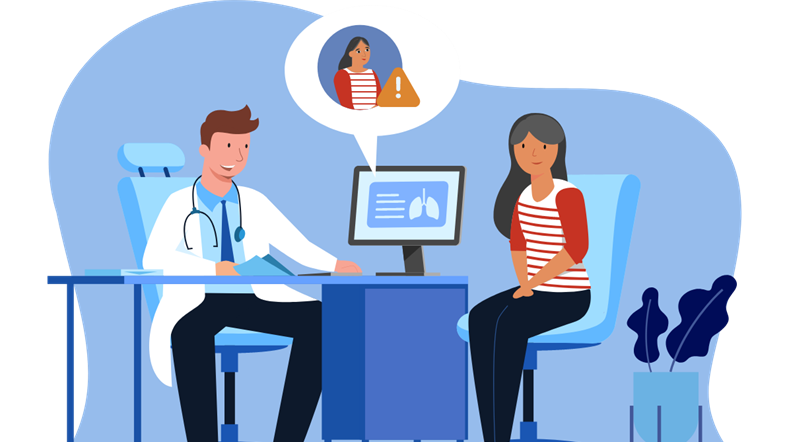
Boost for TNO facilities for sustainable mobility, bio-based construction and AI
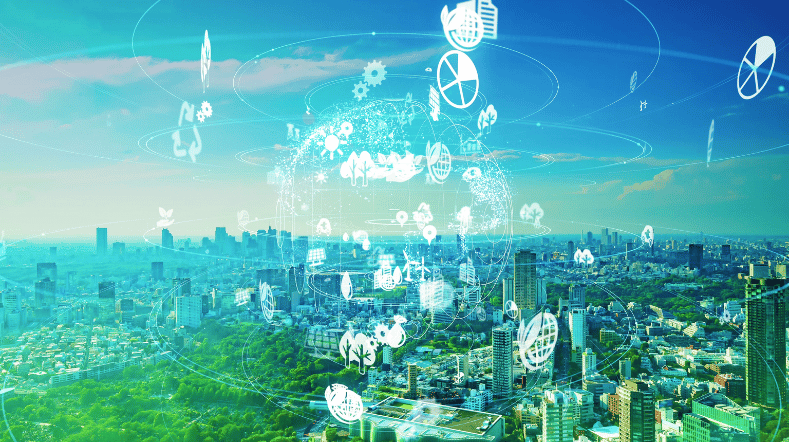
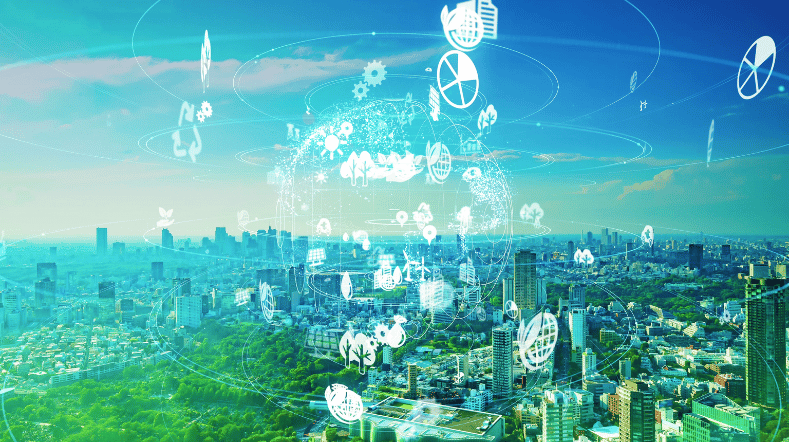
GPT-NL boosts Dutch AI autonomy, knowledge, and technology
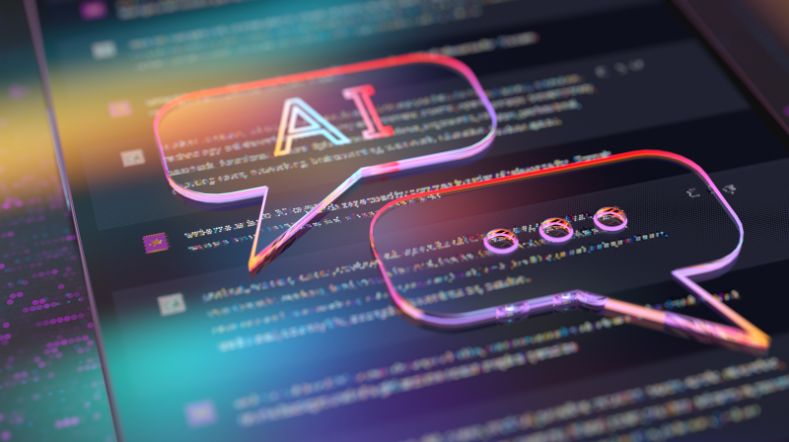
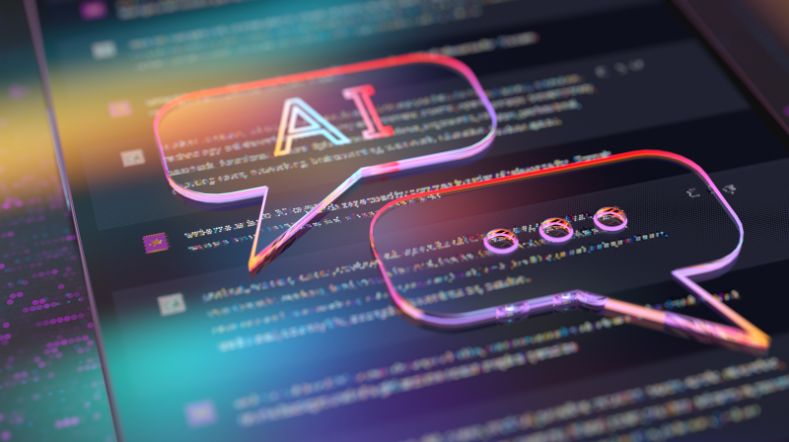
The Netherlands starts realisation GPT-NL, its own open AI-language model
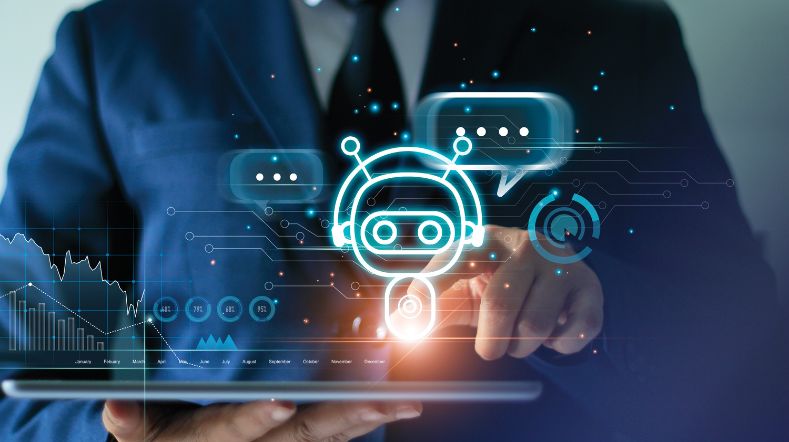
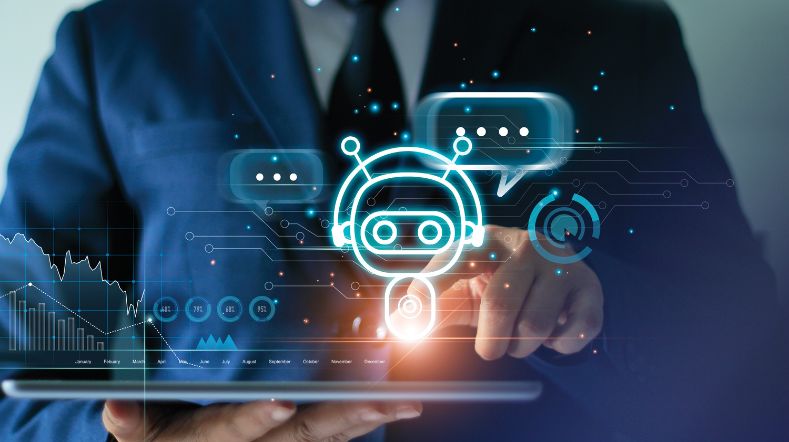
New AI Lab for effective and responsible oversight
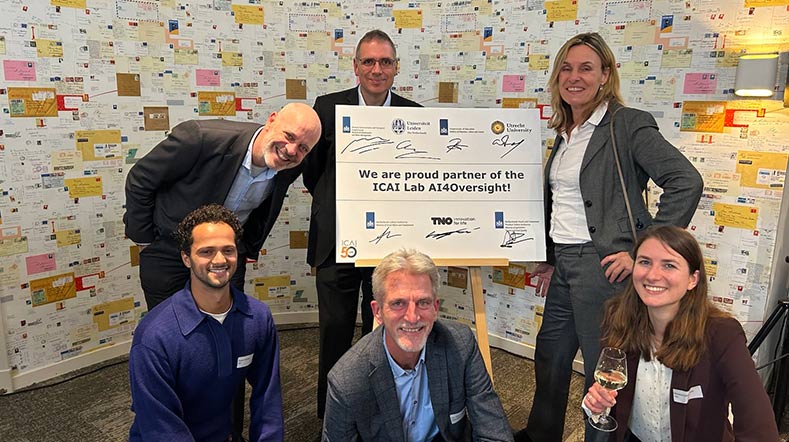
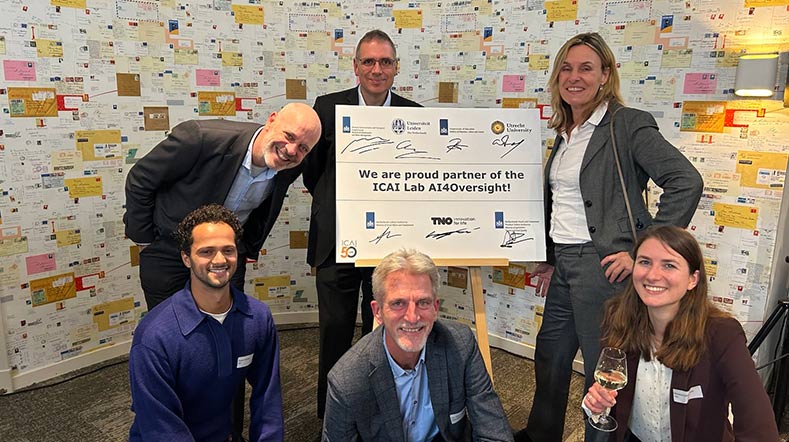