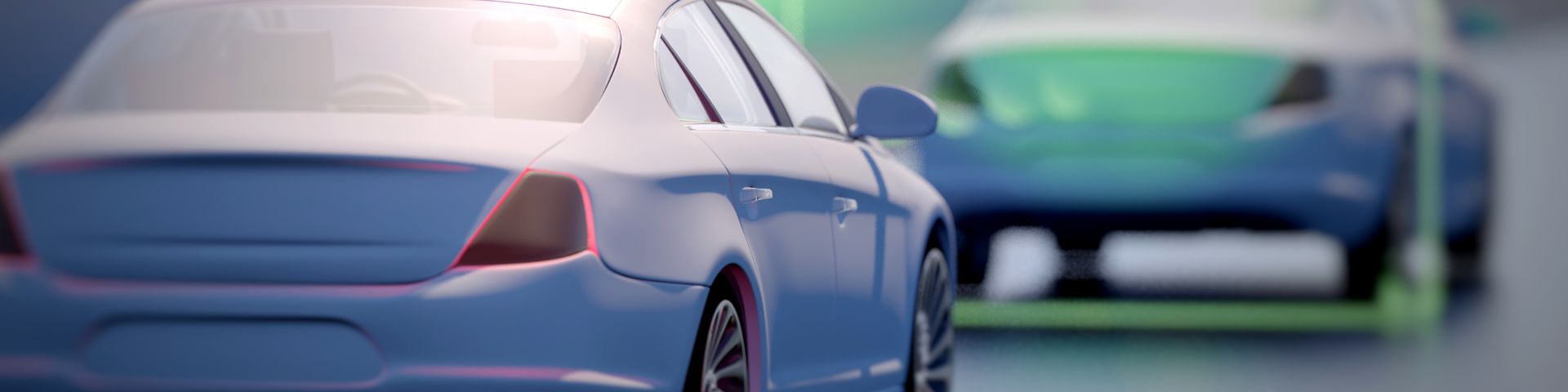
Context-aware motion planning crucial for future of automated driving
Explainability and traceability of AI-based software are crucial elements for the safe deployment and successful certification of automated vehicles. TNO’s hybrid AI approach enhances the reasoning capabilities of self-driving systems by incorporating robust, context-aware motion planning. Experts Chris van der Ploeg and Jochem Brouwer explain how this technology is vital for the future of automated driving.
The introduction of highly automated vehicles on public roads may improve safety and comfort, although their success will largely depend on social acceptance. This requires context-aware motion planning methods that provide safe, proactive, and comfortable trajectories, which are risk-averse, consider predictions about other road users, and comply with traffic rules, social norms, and contextual information. But what exactly is context-aware motion planning?
Meet the expert - Join one or more free and online sessions
What are the milestones on the path to safe automated driving? Join one or more free online sessions and meet the experts.
Understanding the driving environment
An expert on this topic is Chris van der Ploeg, a Research Scientist at TNO. ‘Current ADAS systems, such as Adaptive Cruise Control or Lane Keeping Assist, are purely reactive systems that rely solely on sensory perception. Situational awareness, however, involves understanding the driving environment and considering potential risks. For example, if you’re driving into a densely populated area and see a ball rolling onto the road, current ADAS systems would recognise the object and brake to avoid a collision. With reasoning, we can add context: in this type of environment, there may be many pedestrians, and the fact that it’s a ball increases the likelihood that someone might run onto the road to retrieve it. Situational awareness focuses on the combination of understanding the situation and acting accordingly; what conclusions can I draw from the situational factors, and how should I respond to them?’
Hybrid AI provides reasoning power
TNO’s context-aware motion planning concept is based on its Hybrid AI approach, explains Jochem Brouwer, Consultant for Automated Driving at TNO. ‘We are seeing a trend, especially in the US, that companies are using end-to-end deep learning to try to solve current challenges with automated driving.’
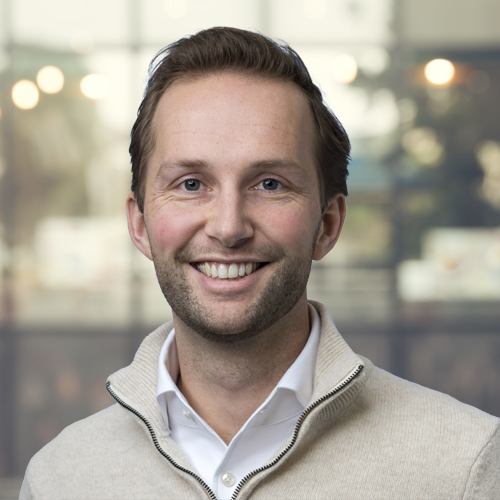
‘The idea is that, with enough data, the system can learn anything. However, if the data don’t include a rare and very specific traffic situation, the system lacks context and might respond undesirably.’
The video of a Waymo vehicle driving behind a trailer loaded with a tree is a good example. Jochem: ‘These vehicles are, on the one hand, trained to follow other road users. On the other hand, when a tree is observed, the vehicle is trained to drive around it, since it expects that a tree is in all cases a static object. If the training data does not show a situation in which a tree is being transported, the vehicle doesn’t recognise the situation. Without situational comprehension or context-awareness, solving such cases is a tedious, if not impossible task. The main challenge of this engineering approach is its scalability because you need to define each specific situation. That’s why TNO advocates a Hybrid AI approach, which still relies heavily on data but combines it with an approach to add reasoning power.’
Sense, comprehend, predict, plan, act
TNO has successfully demonstrated the added value of its Hybrid AI concept with context-aware motion planning in several use cases, of which some were demonstrated during the 2023 Automotive Week.
Chris van der Ploeg explains, ‘Our concept employs a comprehensive sense-comprehend-predict-plan-act process. A sensor fusion perception system provides observations of the traffic situation and objects. To comprehend the situation, we use software tools to determine how the current observed situation aligns with different elements of a predefined model of the driving environment. The understanding of the combination of driving environment elements is then used as optimisation parameter for the motion planner, making it both adaptive and context-aware.’
Introducing the knowledge graph
The so-called knowledge graph is the central element and the "brain" of the AI system, continuously monitoring perception and meaning to establish relationships between objects and elementary driving rules applicable in each situation.
Van der Ploeg elaborates, ‘Based on the observed objects, the knowledge graph adds a complete set of relationships and expected behaviours with respect to these objects, leading to a decision of which driving rules apply to the situation. Furthermore, the knowledge graph reasons about the priority of the applicable driving rules. Based on these decisions and the most critical specifications, the driving rules are translated into risk fields. The vehicle then plans a path where it avoids high-risk areas and therefore moves safely through traffic.’
‘For instance, if the vehicle encounters a box on the road and there is an oncoming car, but there’s enough space to overtake safely, the system could determine that it’s safe to overtake the box and continue. However, if the road has a solid line, overtaking might not be allowed, so traffic rules come into play. The priority could change if, for example, the box is a person—then, to prevent an accident, crossing the line and moving to the other side of the road might be necessary. In this case, safety could take precedence over traffic rules. And then there’s the question of driving comfort and social norms.’
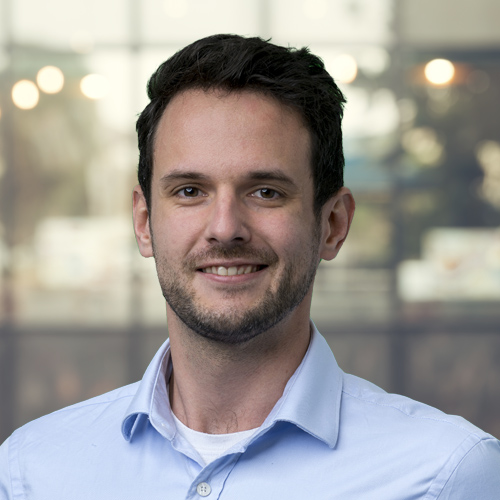
‘Do we want to strictly obey every traffic sign, potentially causing uncomfortable or even dangerous driving behaviour, instead of smoothly integrating with the flow of traffic and being a socially responsible driver?’
Human drivers can easily decide in these situations based on their comprehension of the different priorities. In TNO’s approach this human knowledge is now in fact captured in the knowledge graph.
Proceed with caution
The context-awareness concept was recently enhanced with additional insights derived from Chris van der Ploeg's PhD research. ‘Part of my research focused on situational awareness, specifically on the predictability of risks in certain traffic situations where objects or people might suddenly appear. We identified specific scenarios: the first being a busy, cluttered intersection in an urban area; the second involves a motorbike hidden behind a truck while overtaking, creating an unclear situation; and finally, a pedestrian suddenly appearing from behind a parked vehicle while attempting to cross the road. Most human drivers understand the potential danger in these situations and anticipate by slowing down. Automated vehicles should also learn to comprehend these high-risk scenarios and proceed with caution. We’ve also translated these potential occlusions into risk fields, providing valuable input for motion planning software to enhance the realistic complexity of the environment for automated vehicles.’
Explainability is key
TNO believes that context-aware motion planning could have prevented many incidents and accidents involving self-driving vehicles in the US, where deep learning AI is predominantly used. Europe, however, is taking a more cautious, step-by-step approach to the introduction of automated driving, as Jochem Brouwer explains. ‘Before you can drive an automated vehicle on European roads, you must prove that it is safe in all situations. One of the problems with deep learning AI is explainability: it’s extremely difficult to demonstrate cause and effect when a system bases its behaviour on non-specific data. You can run simulations, but that doesn’t necessarily make the vehicle’s driving behaviour more predictable. With a hybrid approach, we add reasoning to AI, making the transition from ‘sense’ to ‘act’ transparent. This concept provides clarity on the cause and effect of the vehicle’s driving behaviour at all times, even in very subtle cases. In Europe, this is a crucial element for successfully certifying and safely deploying automated vehicles. At TNO, we firmly believe that this concept can positively impact a safe transition towards automated driving.’
Get inspired
Getting on board with autonomous transport? Five things you need to know
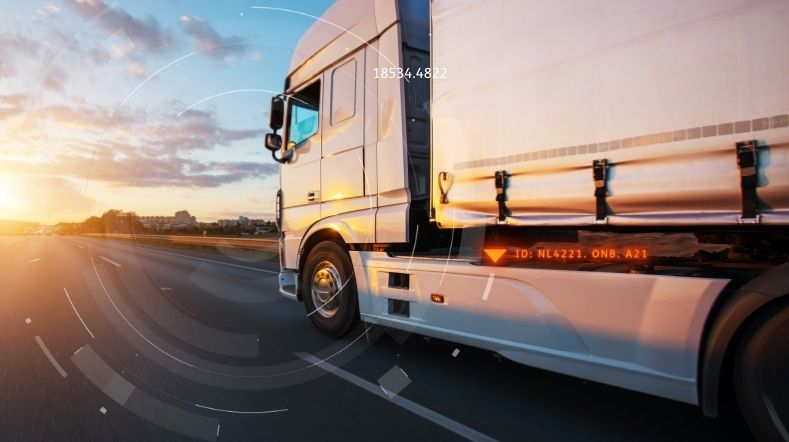
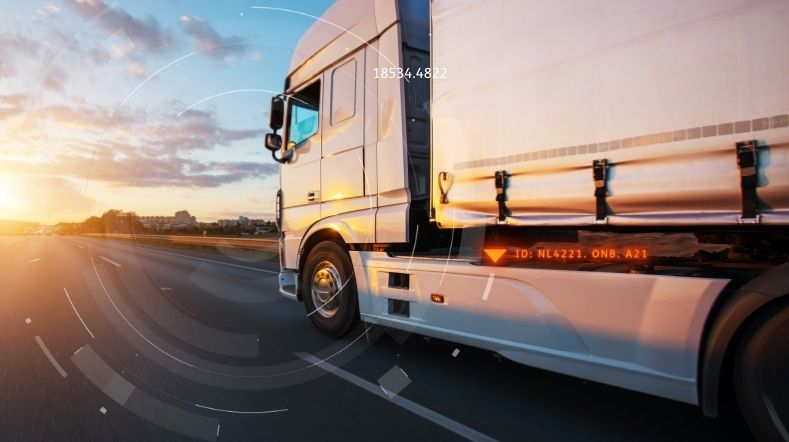
Connected mobility
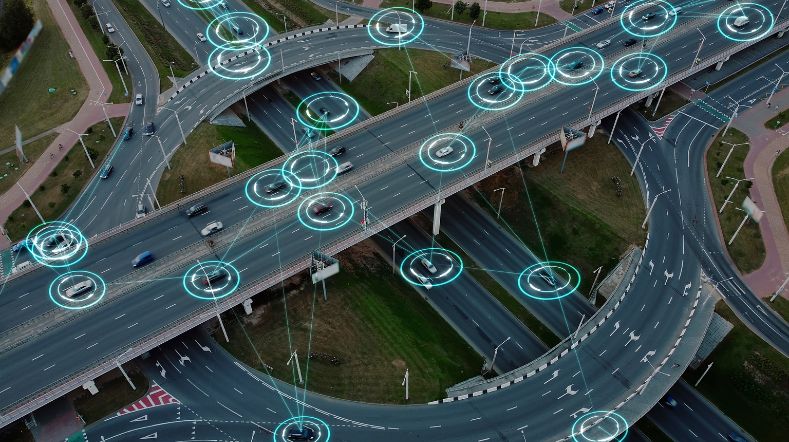
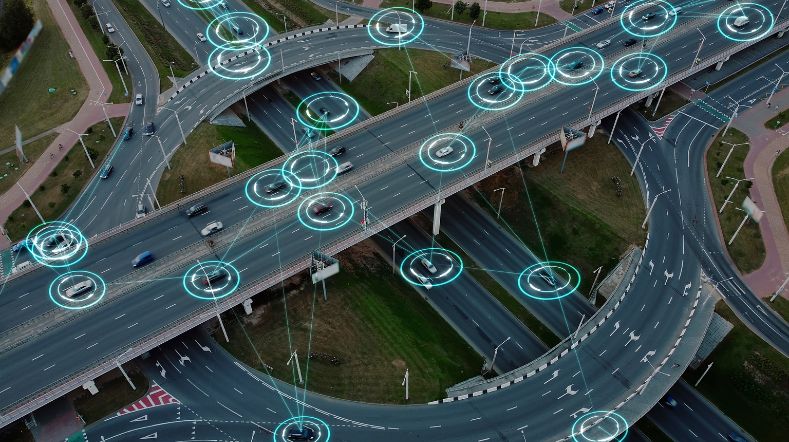
Working on safer self-driving cars
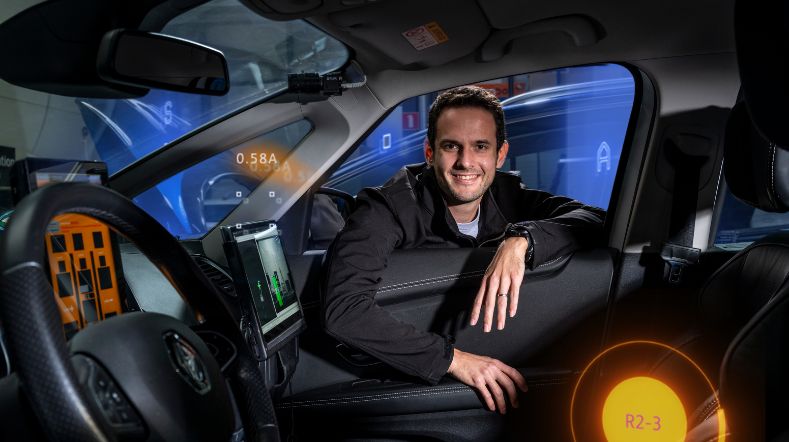
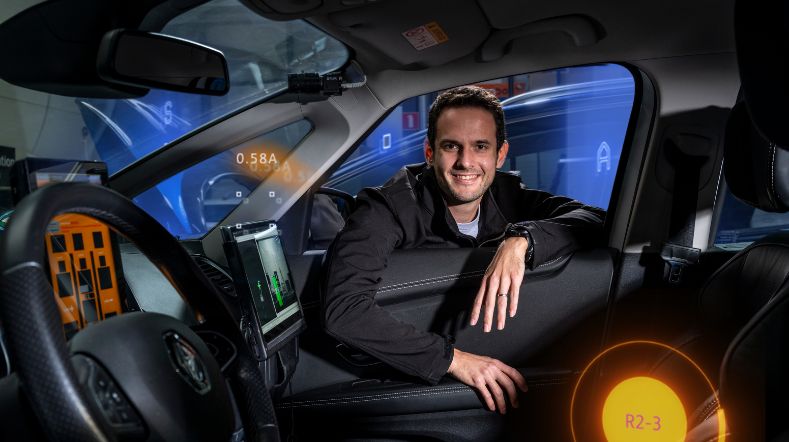
Goodyear and TNO further integrate tyre and vehicle technology to mitigate crashes in adverse weather
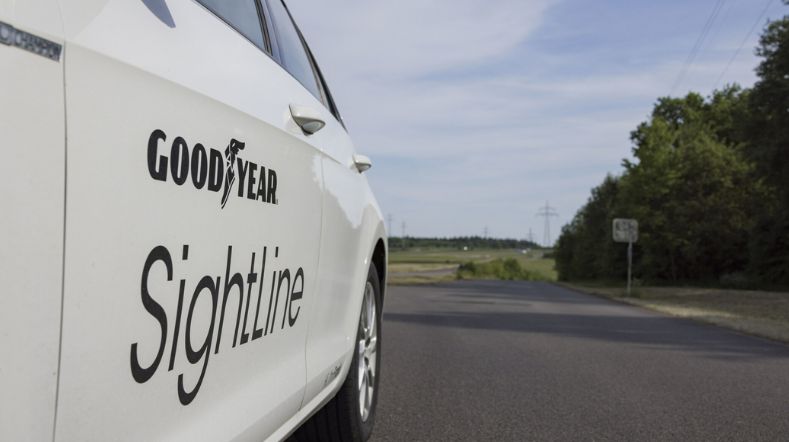
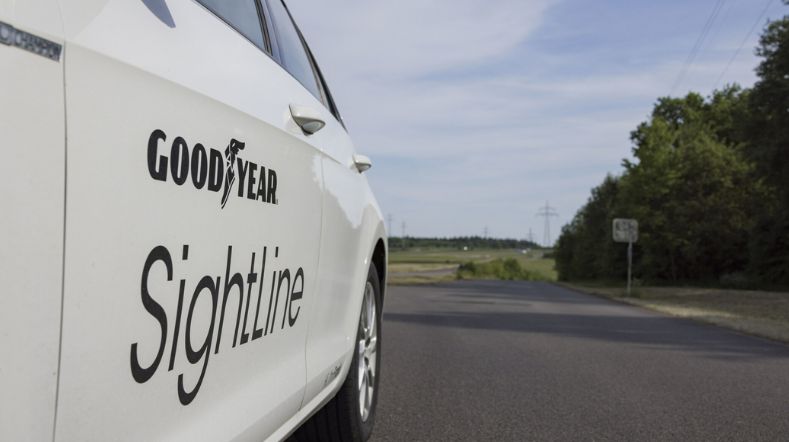
TNO CEYAS offers logistics partners advantage yard automation
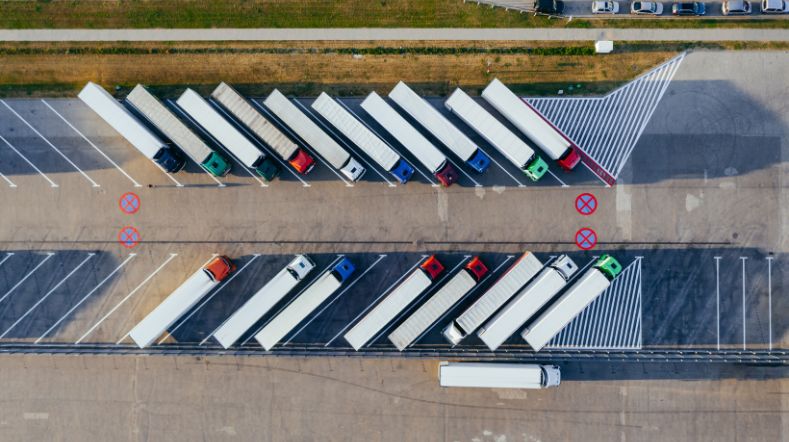
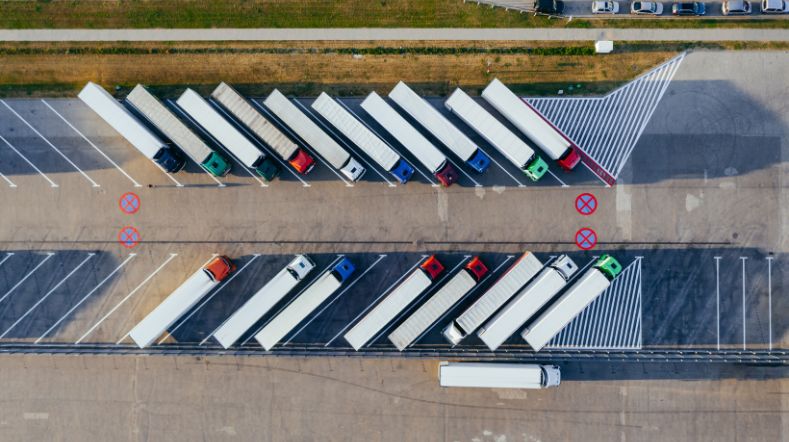