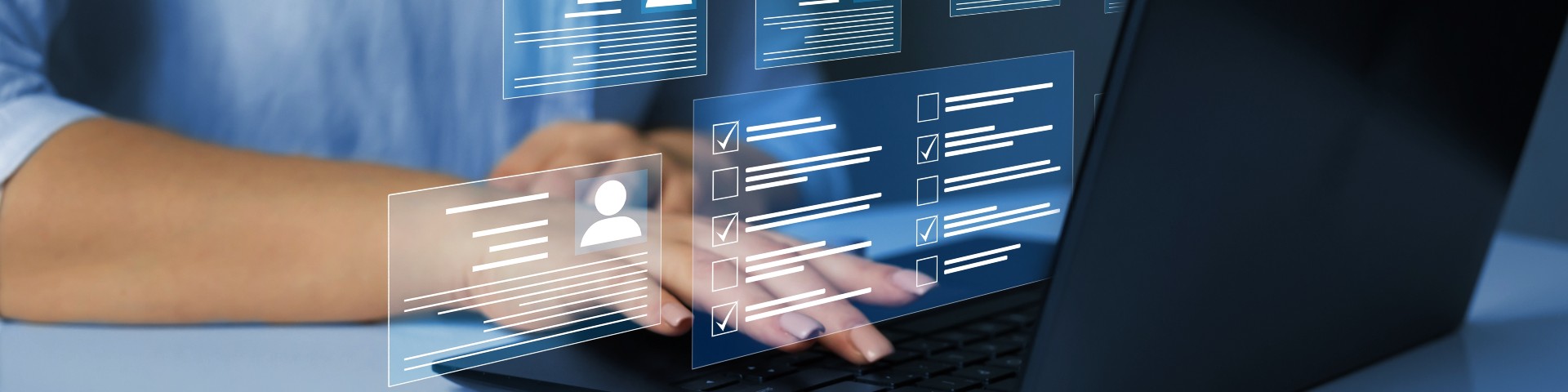
Synthetic data generation: secure learning from personal data
Personal data from patients, citizens, or customers can be valuable and instructive for organisations, but the use of such data often raises privacy issues. Synthetic data may be the answer to this problem. These artificially generated data do not consist of real people, but they can be used for analysis and prediction.
Using and enriching personal data creates new insights and innovative solutions that can contribute to societal solutions. Examples are personalised care or more effective fraud prevention. But how do you handle personal data securely and without violating privacy?
At TNO, we’re working on various privacy-enhancing technologies, such as multi-party computation (MPC), federated learning, and synthetic data generation (SDG).
SDG methods create an entirely new, artificial dataset that can be used instead of the original, privacy-sensitive data. Synthetic data accurately simulate real-world connections, making them suitable for a variety of analytics and AI techniques. Because they do not contain any real personal information, these artificial data can provide an alternative approach.
How SDG works
Synthetic data are generated by first creating a model from personal data, which can then be used to generate new, simulated data. Such a model is created using Artificial Intelligence (AI), Machine Learning (ML), or statistical methods to determine what information from the original data is to be included.
This enables you to determine the properties of variables, for example, that an age cannot be negative, or that nursing home residents have a high average age. You can also define the relationships between variables, for example that men are, on average, taller than women.
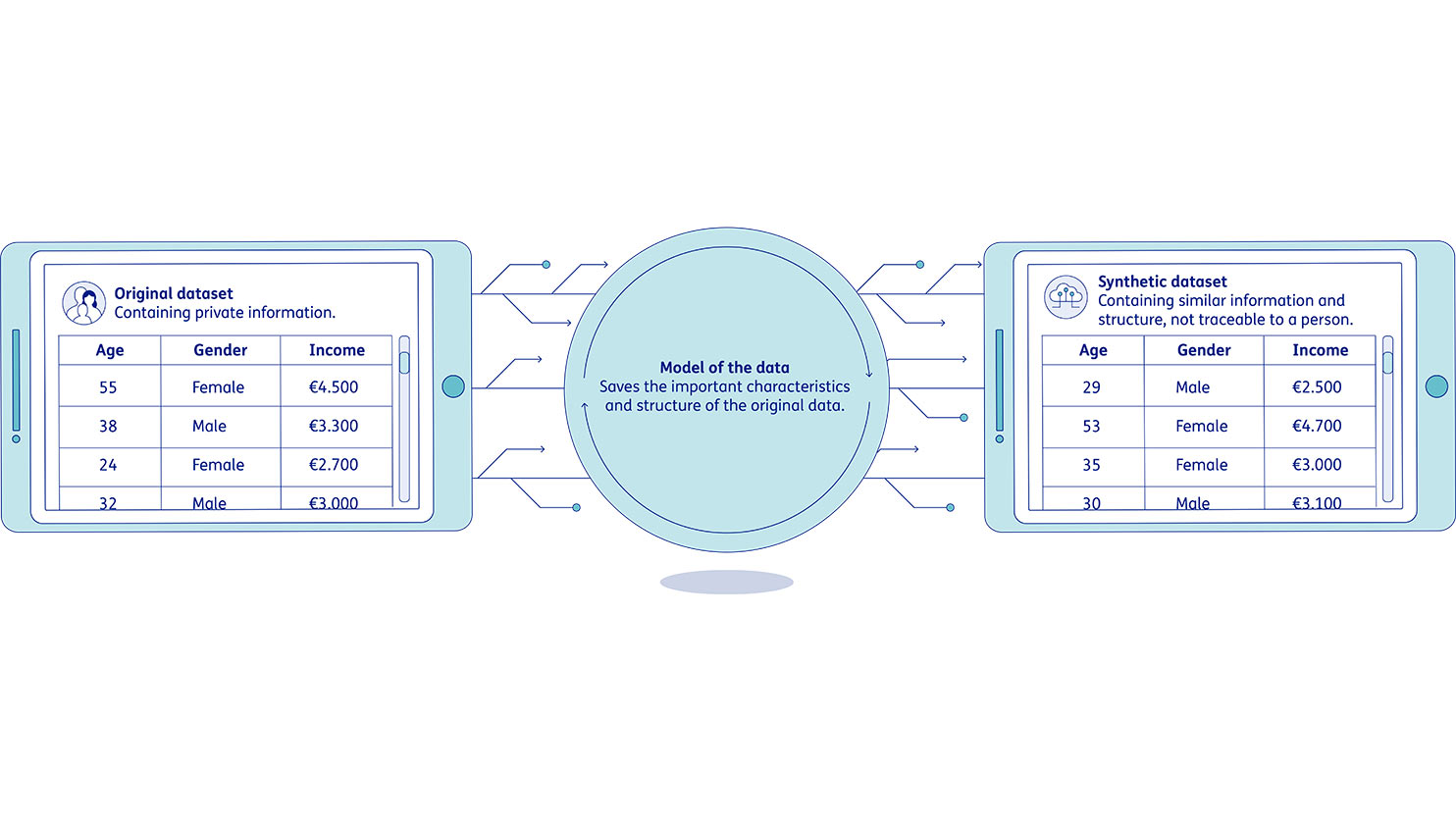
The visual explains how synthetic data generation works. On the left-hand side, you can see the original data with private information about age, gender, and income. A model is generated from that data, where the important features and structure of the data remain intact. The right-hand side of the image shows the synthetic data that came from the model. This is a dataset with information that is no longer traceable to a specific individual.
Greater transparency
Synthetic data are mainly used for analyses that cannot be performed with original, personal data for privacy reasons.
SDG therefore enables secure sharing of data with external parties to produce new insights. It also enables organisations to be more transparent and makes knowledge-building with data easier and more accessible.
SDG will make it much easier to conduct research using data from patients, private individuals, users, and customers. This can help optimise patient care, increase the efficiency of local authorities, or provide better products and services for consumers, for example.
Synthetic data against money laundering
An interesting application of SDG is detecting money laundering. Transaction data from multiple banks are needed to detect illicit money flows. But such data exchanges conflict with privacy laws and concerns about customer and bank privacy.
To use privacy enhancing technologies for securely detecting money laundering transactions, the Alliance of Privacy Preserving Detection of Financial Crime (APP-DFC) (opens in a new window or tab) (refers to a different website) has been established. For this consortium of Rabobank, ABN AMRO, TMNL, Volksbank, CWI, and TNO, we developed a synthetic transaction generator.
Synthetic transactions and accounts mimic properties of sensitive transaction data. This enables us to share properties of the data without revealing information about the actual transactions.
TNO is also working to develop a synthetic transaction network based on data from multiple banks without them having to exchange data. For this purpose, we use a unique combination of SDG and MPC.
What opportunities does SDG offer your organisation?
Although SDG is a relatively new solution to the conflict between knowledge-building and privacy, TNO offers a research group with extensive experience in synthetic data in various domains.
For example, we have now synthesised tables, transaction networks, and texts. In addition, TNO distinguishes itself by continuously researching and developing new methods for SDG, while prioritising both privacy preservation and information quality.
At TNO, we’re looking for partners for whom existing SDG methods are insufficient, either because suitable methods do not yet exist for their type of data, or because the quality of the synthesised data is insufficient.
Evaluation methods for privacy and data quality may also be underdeveloped. Don’t hesitate to contact us and find out whether synthetic data generation can provide a solution for your organisation.
Get inspired
Digitalisation and sustainability: how AI can help
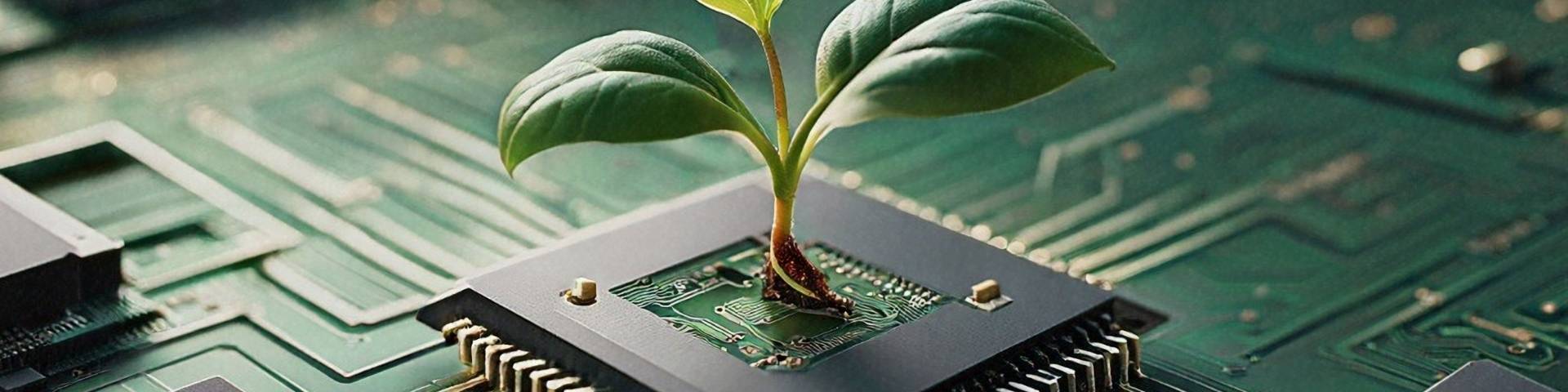
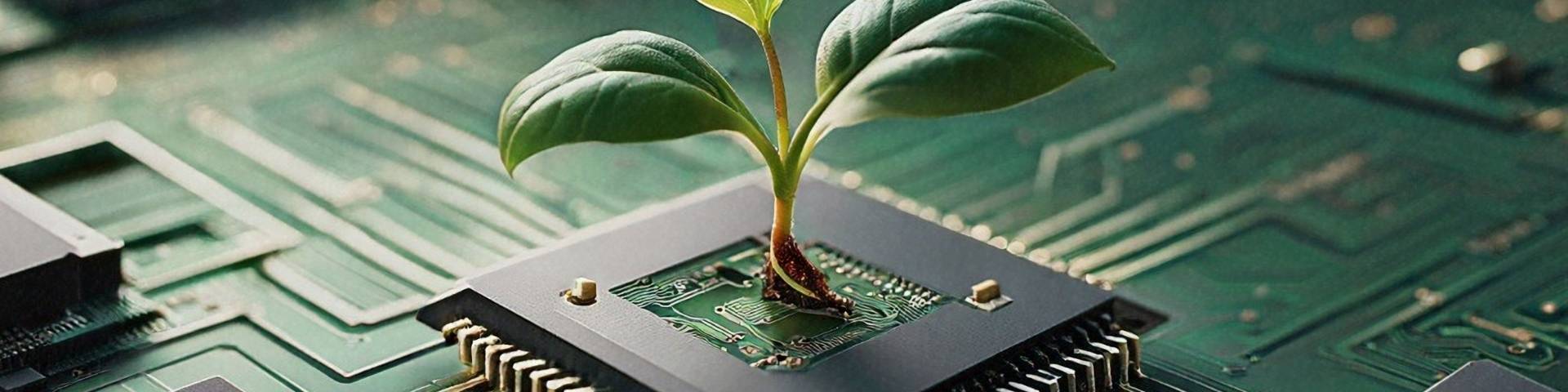
Time setter story: Kallol Das
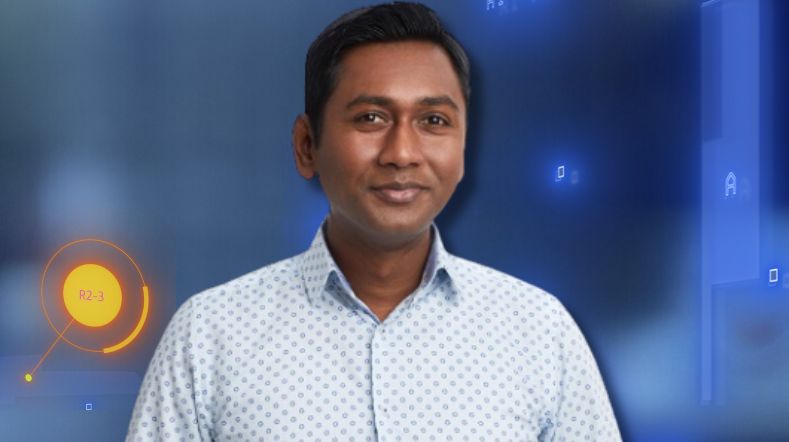
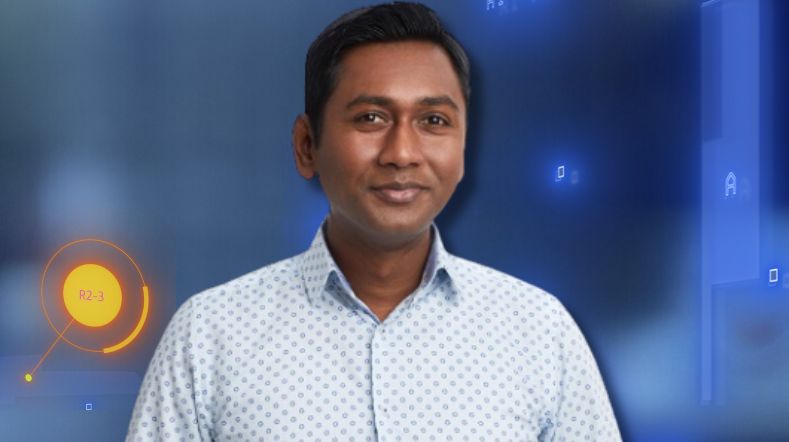
Digital Product Passport
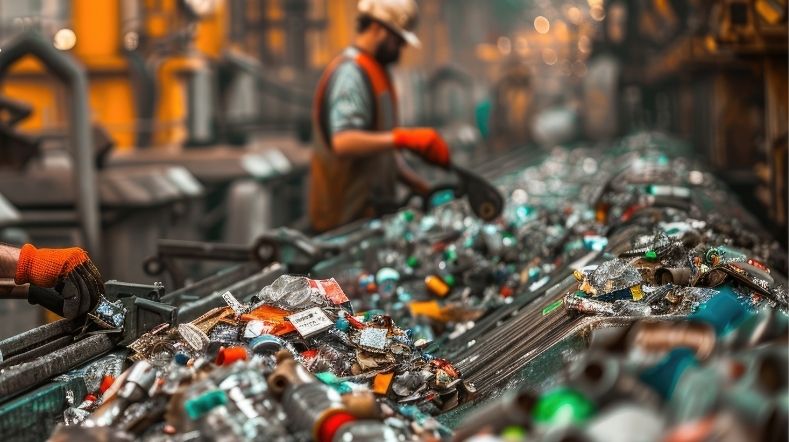
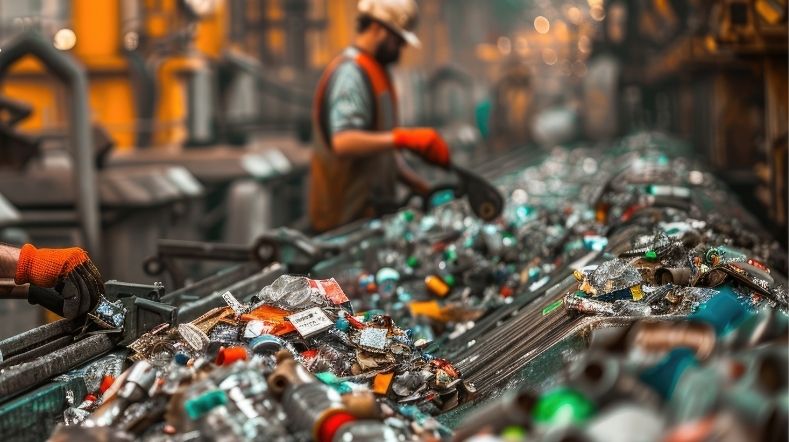
Time setter story: Annemieke Kips
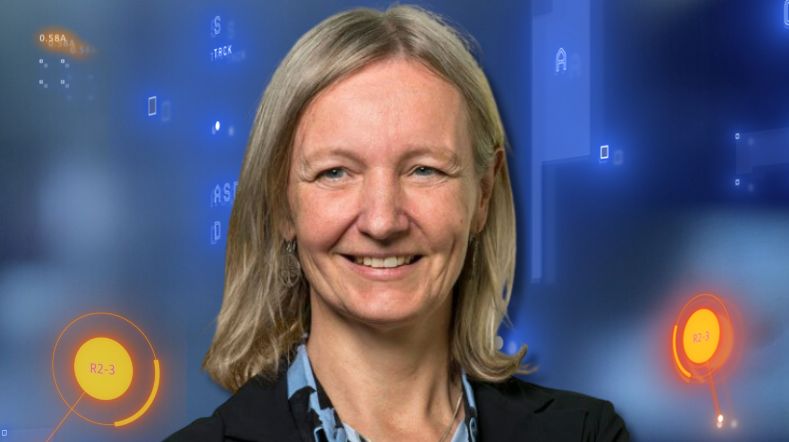
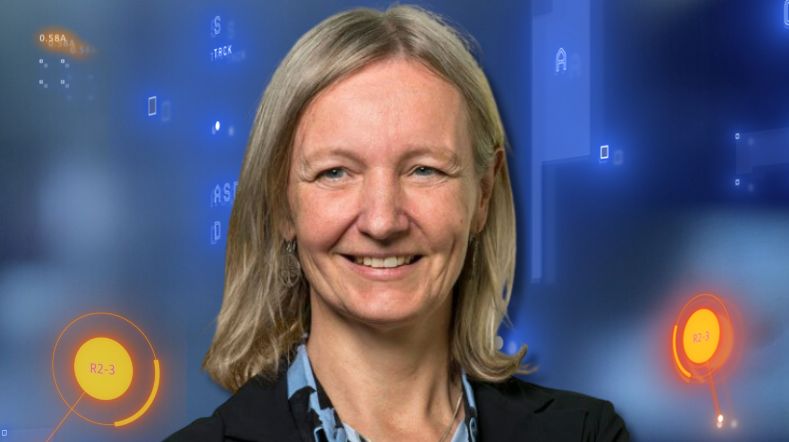
Time setter story: Belma Turkovic
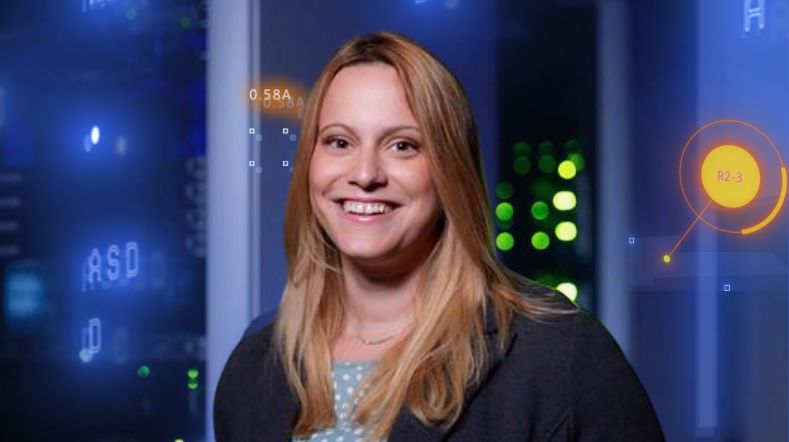
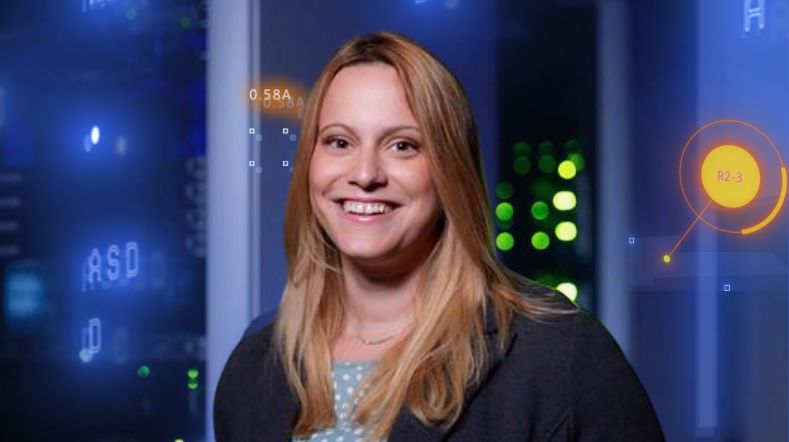